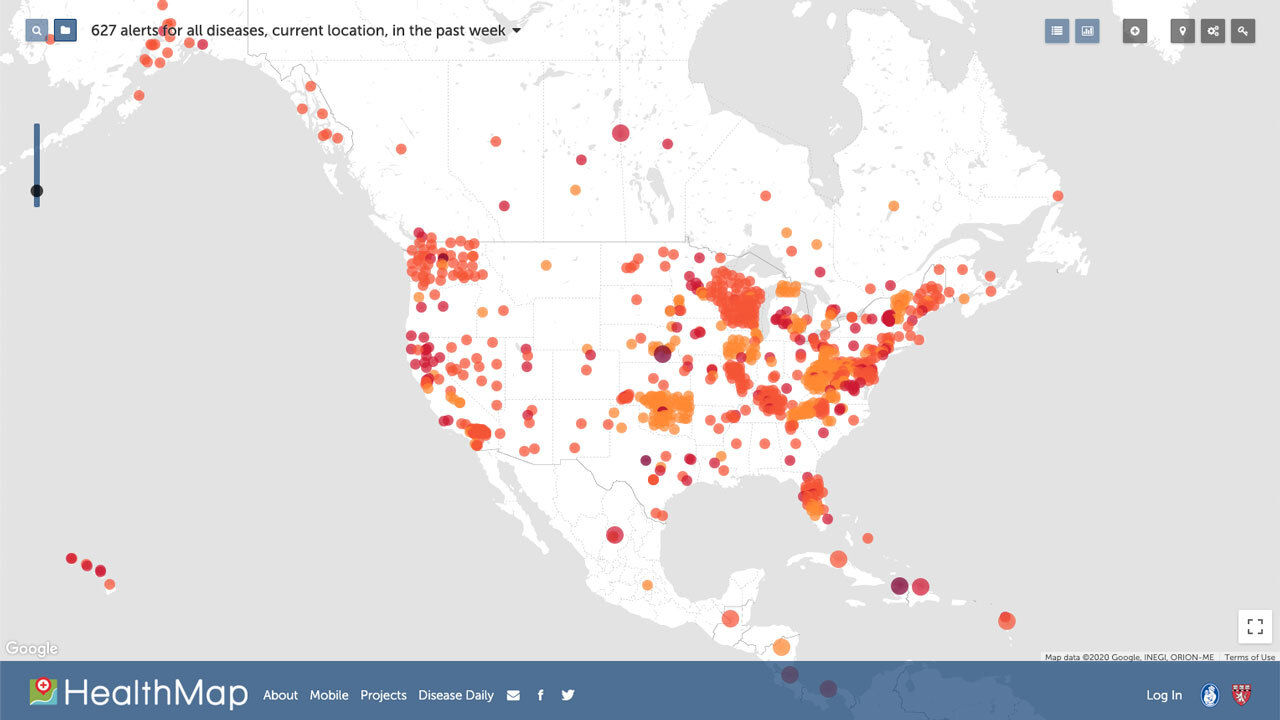
The international alarm about the COVID-19 pandemic was sounded first not by a human, but by a computer. HealthMap, a website run by Boston Children's Hospital, uses artificial intelligence (AI) to scan social media, news reports, internet search queries, and other information streams for signs of disease outbreaks. On 30 December 2019, the data-mining program spotted a news report of a new type of pneumonia in Wuhan, China. The one-line email bulletin noted that seven people were in critical condition and rated the urgency at three on a scale of five.
Humans weren't far behind. Colleagues in Taiwan had already alerted Marjorie Pollack, a medical epidemiologist in New York City, to chatter on Weibo, a social media site in China, that reminded her of the 2003 outbreak of severe acute respiratory syndrome (SARS), which spread to dozens of countries and killed 774. "It fit all of the been there, done that déjà vu for SARS," Pollack says. Less than 1 hour after the HealthMap alert, she posted a more detailed notice to the Program for Monitoring Emerging Diseases, a list server with 85,000 subscribers for which she is a deputy editor.
But the early alarm from HeathMap underscores the potential of AI, or machine learning, to keep watch for contagion. As the COVID-19 pandemic continues to spread around the globe, AI researchers are teaming with tech companies to build automated tracking systems that will mine vast amounts of data, from social media and traditional news, for signs of new outbreaks. AI is no substitute for traditional public health monitoring, cautions Matthew Biggerstaff, an epidemiologist with the U.S. Centers for Disease Control and Prevention (CDC). "This should be viewed as one tool in the toolbox," he says. But it can fulfill a need, says Elad Yom-Tov, a computer scientist with Microsoft who has worked with public health officials in the United Kingdom. "There's such a wealth of data, we will need some sort of tool to make sense of those data, and to me that tool is machine learning."
Well before COVID-19 hit, CDC began an annual competition to most accurately predict the severity and spread of influenza across the United States. The competition, started in 2013, receives dozens of entries each year; Biggerstaff says roughly half involve machine learning algorithms, which learn to spot correlations as they are "trained" on vast data sets. For example, Roni Rosenfeld, a computer scientist at Carnegie Mellon University, and colleagues have won the competition five times with algorithms that mine data on, among other things, Google searches, Twitter posts, Wikipedia page views, and visits to the CDC website.
Many of teams involved in the flu challenge have now pivoted to tracking COVID-19. They are applying AI in two ways. It can strive to spot the first signs of a new disease or outbreak, just as HealthMap did. That requires the algorithms to look for poorly defined signals in a sea of noise, a challenge on which a well-trained human may still hold the upper hand, Pollack says.
AI can also be used to assess the current state of an epidemic—so-called now-casting. The Carnegie Mellon team aims to now-cast COVID-19 across the United States, using data collected through pop-up symptom surveys by Google and Facebook, Google search data, and other sources in order to predict local demand for intensive care beds and ventilators 4 weeks into the future, Rosenfeld says. "We're trying to develop a tool for policymakers so that they can fine-tune their social distancing restrictions to not overwhelm their hospital resources."
Although automated, AI systems are still labor intensive, notes Rozita Dara, a computer scientist at the University of Guelph who has tracked avian influenza and is turning to COVID-19. "By the time you get to AI, it's the easy part," she says. To train a program to scan Twitter, for example, researchers must first feed it examples of relevant tweets, selected by weeding through Twitter for many hours, Dara says. AI may also struggle in a rapidly evolving pandemic, where correlations between online behavior and illness can shift, says Jeffrey Shaman, an epidemiologist at Columbia University.
AI has misfired before. From 2009 to 2015, Google ran an effort called Google Flu Trends (now part of HealthMap's machinery) that mined search query data to track the prevalence of flu in the United States. At first the system did well, correctly predicting CDC tallies roughly 2 weeks ahead of time. However, from 2011 to 2013, it overestimated the prevalence of flu. That failure arose largely because researchers didn't retrain the system as people's search behavior evolved, Yom-Tov says, and it misinterpreted searches for news reports about the flu as signs of infection.
"I don't think it's an inherent problem with the field," Yom-Tov adds. "It's something that we've learned from." In fact, he and colleagues from University College London recently posted a paper to the arXiv preprint server showing they could correct for that media-related bias.
Officials in nations that struggle to provide adequate testing for the new coronavirus, such as the United States, might be tempted to use automated surveillance systems instead. Biggerstaff says that would be a mistake: "I don't think this can replace testing in any way." In particular, he says, when the flu re-emerges this fall, direct testing will be necessary to distinguish outbreaks of influenza from COVID-19. But AI might help policymakers direct more testing to hot spots. "The hope is that you would actually have the two working together," says John Brownstein, an epidemiologist at Boston Children's who co-founded HealthMap in 2006.
Some researchers question whether AI systems will be ready in time to help with the COVID-19 pandemic. "AI will not be as useful for COVID as it is for the next pandemic," says Dara, who expects it will take about 6 months to develop her system for tracking the disease. Still, data mining and machine learning in epidemiology seem here to stay. Pollack, who sounded the alarm about COVID-19 the old-fashioned way, says she, too, is working on an AI program to help scan Twitter for mentions of the disease.
COVID-19 Update: The connection between local and global issues–the Pulitzer Center's long standing mantra–has, sadly, never been more evident. We are uniquely positioned to serve the journalists, news media organizations, schools, and universities we partner with by continuing to advance our core mission: enabling great journalism and education about underreported and systemic issues that resonate now–and continue to have relevance in times ahead. We believe that this is a moment for decisive action. Learn more about the steps we are taking.